Detailed Program
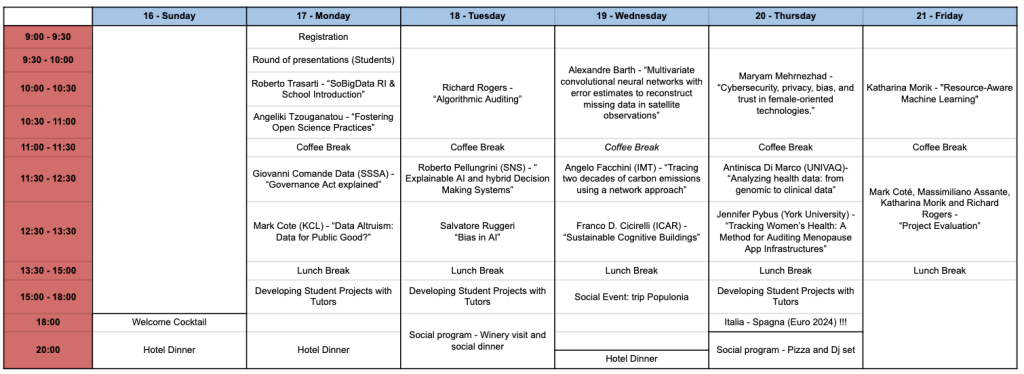
Fostering Open Science Practices throughout the Research Lifecycle
(Angeliki Tzouganatou)
In this talk, we will delve into the pivotal role of Open Science beyond publishing, emphasizing its importance throughout the entire research cycle for fostering innovation, transparency, and inclusivity. We will explore how open practices can democratize science in the digital ecosystem, promoting equitable access to information and a culture of collaboration fostering community governance. The talk will highlight and demonstrate OpenAIRE's comprehensive suite of services, putting these principles into practice. Join us to discover how Open Science practices are forging a more accessible and equitable future within the digital research landscape.
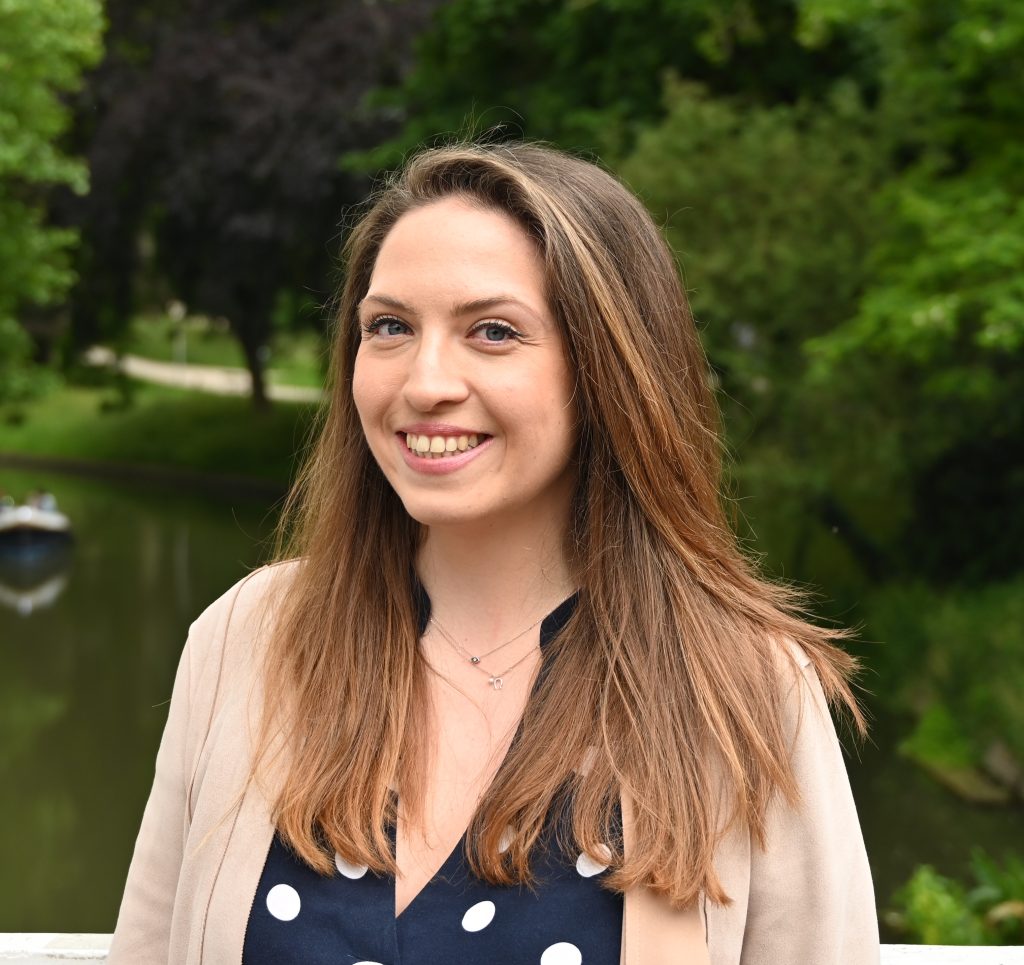
Data Governance Act explained (Giovanni Comandè)

Full Professor of Private Comparative Law at Scuola Superiore S. Anna Pisa, Italy. PhD. SSSA, LLM Harvard Law School, Founder and Director of the LIDER-LAB (www.lider-lab.it). Attorney at law (Pisa since 1995); (New York Bar since 1997). Mediator mediation trainer. He has taught in and visited numerous universities in the world. Scientific director of many research projects funded by MIUR, CNR, ESF, EU, the Canadian Ministry of Foreign Affairs Public Administrations, public and private companies and organizations. Main current projects: LEADs -Legality Attentive Data Scientists (PI) H2020-MSCA-ITN-2020; SoBigData++: European Integrated Infrastructure for Social Mining and Big Data Analytics; INFRAIA-H2020-EU.1.4.1.2. (task Leader) – Predictive Jurisprudence, (PI) Scuola Superiore Sant’Anna. Author of 6 monographs, editor of 15 collective works, published more than 200 articles and notes in major law reviews, and chapters to collective publications in Italian, English, French and Spanish.
Data Altruism: Data for Public Good? (Mark Coté)
Data Altruism was granted statutory footing by the Data Governance Act from Sept 2023; it will enable citizens to share their data with greater consent, control, and privacy in a manner that has demonstrable societal good. This talk will outline the technical challenges to building such a new open infrastructure, with a safe user pipeline, and a multi-level workflow for data scientists. It will also examine the social challenges to a paradigm that stands opposite the monetising commercial model of data for service, and explore the steps to building a trusted research environment for community-driven algorithmic co-design. We will present use case scenarios, including the open-source movement of Type 1 diabetics, and an intersectional CV donation project for facilitating the prevention, detection, and management of discrimination in algorithmic hiring.
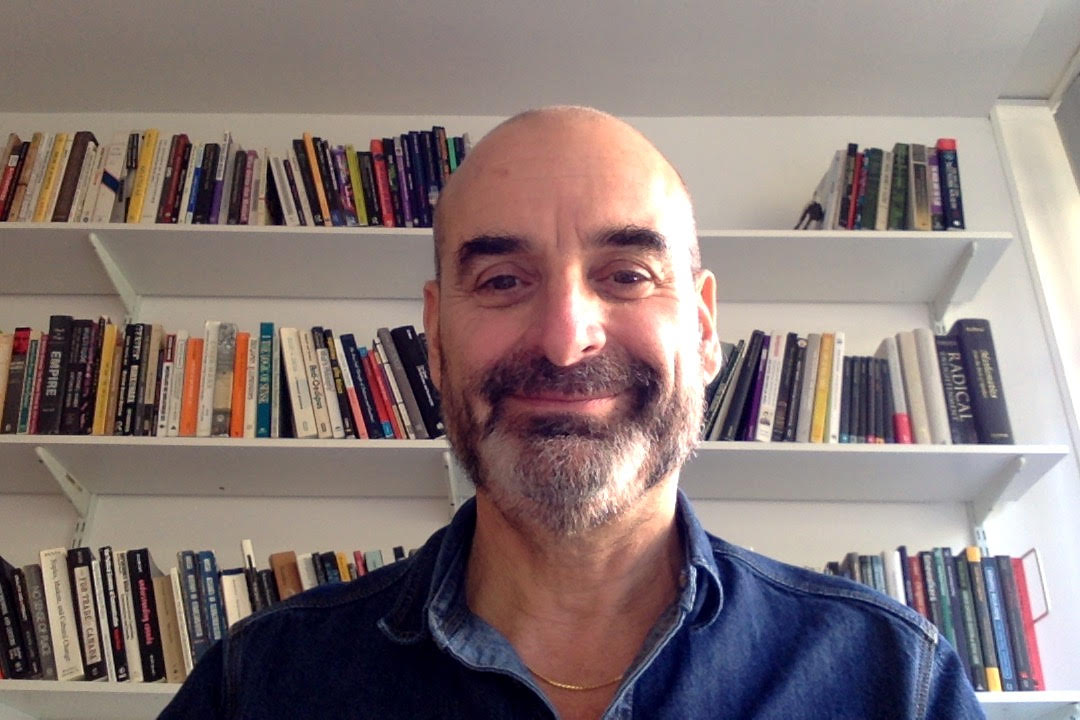
Mark is a research-led academic with a pioneering cross-disciplinary vision that scans both the human and technical object in order to understand the societal dimensions of data, computation and AI. He has been PI or CI on EPSRC, H2020, and AHRC grants valued at more than £10 million. He collaborates with computer scientists in social data analytics and cybersecurity, social scientists and policy experts and legal scholars. He is a PI and Strategic Board member of REPHRAIN, the UK’s national research centre for online harm mitigation and data empowerment, and a PI on SoBigData, the European research infrastructure for social data analytics. He has collaborated with the Open Data Institute, British Library, the University of Pisa, Tactical Tech Collective and many others. He has presented his research at the British Academy, the European Parliament, the International Communication Association, the Royal Society, the Italian National Research Council, and many others. His work has been published widely in leading journals across disciplines including Big Data & Society and the IEEE Computer. His innovative leadership in research-led teaching and curriculum development is demonstrated in the MA Data Culture and Society.
Algorithmic Auditing (Richard Rogers)
Algorithmic auditing is an approach to the study of search engines and social media platforms inherited from the social scientific study of discrimination, where, for example, the same application for housing is submitted, albeit with names that signal differing cultural or ethnic backgrounds. For the study of engines and platforms, it is much broader though discrimination (and by extension content moderation) remains one focal point. The contribution discusses the typical ways in which Google, YouTube, Facebook, Twitter, Instagram and TikTok are ‘audited’, including approaches to the study of self-dealing, platform performer privileging, personalisation, shadow banning, angertainment and others.
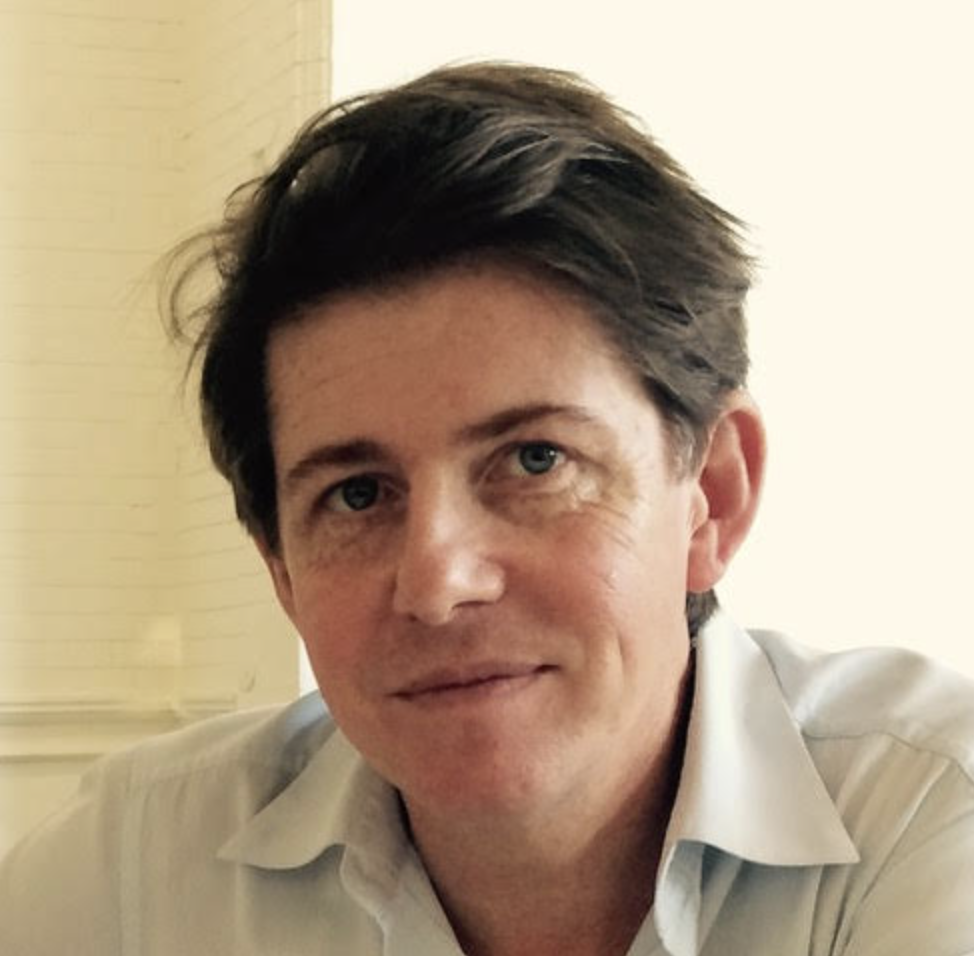
Richard Rogers is Professor of New Media and Digital Culture, Media Studies, University of Amsterdam and Director of the Digital Methods Initiative. He is author of Information Politics on the Web, Digital Methods (both MIT Press) as well as Doing Digital Methods (Sage). He is editor (with Sabine Niederer) of The Politics of Social Media Manipulation and The Propagation of Misinformation in Social Media: A Cross-platform Analysis (both Amsterdam University Press).
Explainable AI and hybrid Decision Making Systems (Roberto Pellungrini)
In this presentation we will introduce the main learning paradigms for Hybrid Decision Making Systems and discuss how Explainable AI can be used to enhance these systems and improve the interaction between humans and AI based systems. We will briefly introduce Explainable AI taxonomy and the methodological foundations for Hybrid Decision Making Systems and understand what kind of explanations can be used for this particular Machine Learning Models and the current state of the art in this field.
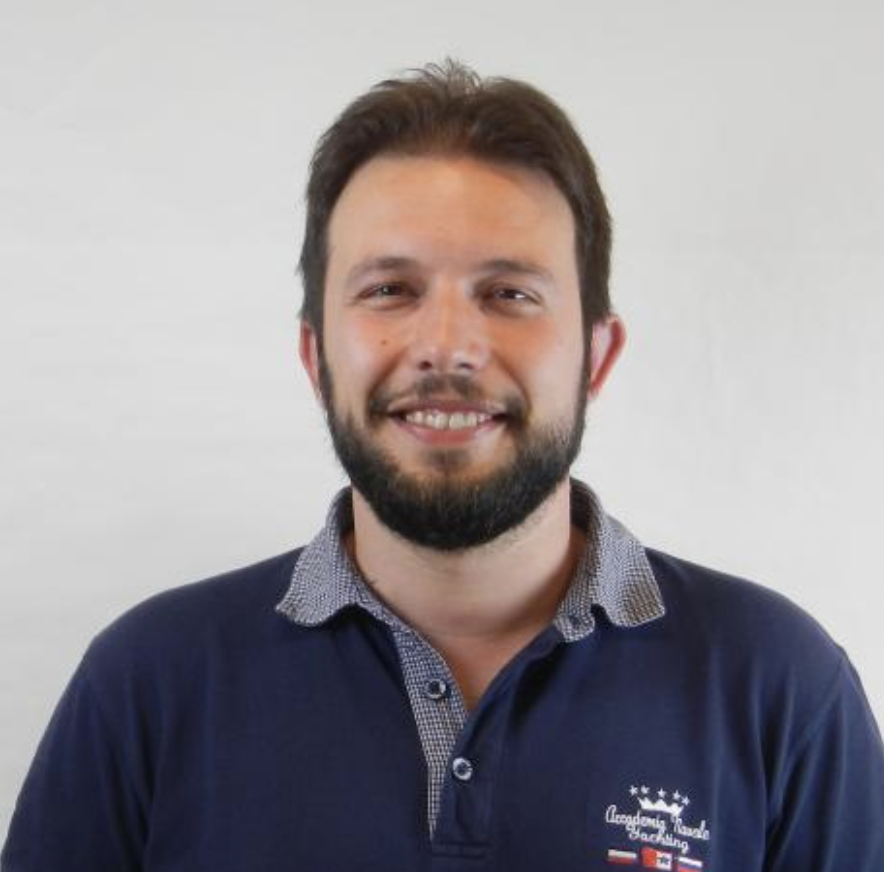
Roberto Pellungrini is a Researcher at Scuola Normale Superiore and a member of the KDD Lab, a joint research initiative of ISTI Institute of CNR, the Department of Computer Science of the University of Pisa and Scuola Normale Superiore. He earned his PhD in Computer Science at The University of Pisa in 2020 with a thesis on Data Privacy. His current research interests are Explainability and Hybrid Decision Making Systems.
Bias and Fairness in AI (Salvatore Ruggeri)
The literature addressing bias and fairness in AI models (fair-AI) is growing at a fast pace, making it difficult for novel researchers and practitioners to have a bird’s-eye view picture of the field. In this talk, we concisely survey the motivation and the state-of-the-art of fair-AI methods and resources, with the aim of providing a multi-disciplinary bird’s-eye guidance. We also present and discuss a few relevant topics which are not sufficiently developed or acknowledged in the literature, which are open for research contributions.

Salvatore Ruggieri is full professor at the Computer Science Department of the University of Pisa, Italy, where he teaches at the Master Program in Data Science and Business Informatics and at the PhD Program in Artificial Intelligence. He holds a Ph.D. in Computer Science (1999), whose thesis has been awarded by the Italian Chapter of EATCS as the best Ph.D. thesis in Theoretical Computer Science. He is a member of the KDD LAB (http://www-kdd.isti.cnr.it/), with research interests focused in the data mining and AI area, including: algorithmic fairness, explainable AI, causality, privacy, languages and systems for modeling the process of knowledge discovery; sequential and parallel classification algorithms; web mining; and applications. He was the coordinator of Enforce, a national FIRB (Italian Fund for Basic Research) young researcher project on Computer science and legal methods for enforcing the personal rights of non-discrimination and privacy in ICT systems (2010-2014, enforce.di.unipi.it). He was the co-program chair of the ACM Conference in Fairness, Accountability and Transparency (FAT*2020), Barcelona, Spain, 27-30 January 2020.
Multivariate convolutional neural networks with error estimates to reconstruct missing data in satellite observations (Alexandre Barth)
Over the past four decades, satellite observations have dramatically increased the coverage for Earth observations and enabled various advances in our understanding and prediction of the Earth systems. For ocean applications, however, satellite observations have data gaps due to e.g. clouds (blocking infrared and visible light from the ocean surface), rain (affecting microwaves) or simply due to the geometry of the satellite tracks. In this presentation we will discuss the use of neural networks to reconstruct the missing data in satellite observations. Contrary to standard image reconstruction (in-painting) with neural networks, most ocean application requires a method to handle missing data (or data with variable accuracy) already in the training phase. Instead of using the commonly used mean square error as a cost function, the neural network (U-Net type of network) is optimized by minimizing the negative log likelihood assuming a Gaussian distribution (characterized by a mean and a variance). As a consequence, the neural network also provides an expected error variance of the reconstructed field (per pixel and per time instance). Neural networks can naturally handle multivariate data (an example will be shown with sea surface temperature, chlorophyll concentration and wind fields). The improvement of this network is demonstrated for sea surface temperature of the Adriatic Sea. Applications with denoising diffusion models to reconstruct missing observations are also shown. Such methods can naturally provide an ensemble of reconstructions where each member is spatially coherent with the scales of variability and with the available data. Rather than providing a single reconstruction, an ensemble of possible reconstructions can be computed, and the ensemble spread reflects the underlying uncertainty.
Alexandre Barth is an Associate professor at Université de Liège and senior research fellow at Fonds de la Recherche Scientifique – FNRS
Tracing two decades of carbon emissions using a network approach
(Angelo Facchini)
Carbon emissions are currently attributed to the producers of goods and services. This approach has been challenged by advocating an attribution criterion based on consumers, i.e. accounting for the carbon embedded into the goods imported by each country. Quantifying the effectiveness of such a consumption-based accounting requires understanding the complex structure of the graph induced by the flows of emissions between world countries. To this aim, we have considered a balanced panel of a hundred countries and constructed the corresponding Carbon Trade Network for each of the past twenty years. Our analysis highlights the tendency of each country to behave either as a `net producer’ – or `net exporter’ – of emissions or as a `net consumer’ – or `net importer’ – of emissions; besides, it reveals the presence of unexpected, positive feedback: despite individual exchanges having become less carbon-intensive, the increasing trade activity has ultimately risen the amount of emissions directed from `net exporters’ towards `net importers’. Adopting a consumption-aware accounting would re-distribute responsibility between these two groups, possibly reducing disparities.
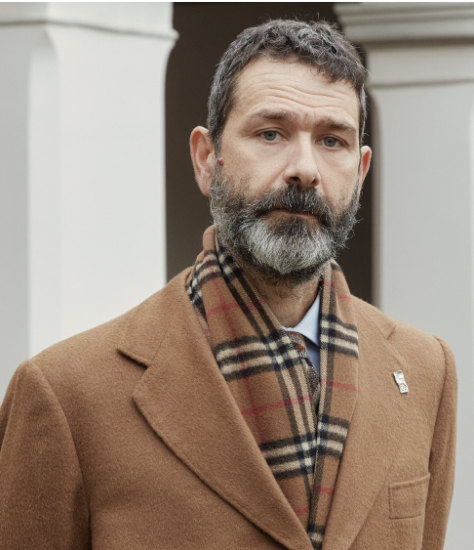
Angelo Facchini is a researcher at IMT Alti Studi Lucca and works on project management on big data, climate change, complex systems , urban development, energy systems, water infrastructures. It is also a consultant for digitalization of water infrastructures: application of big data analytics and complex networks methods, asset management, predictive maintenance. Works on the sustainable urban development, urban metabolism, energy, water and waste flows in Megacities, resilience of urban systems and critical infrastructures, energy use and energy efficiency in cities and in the built environment, energy use in informal settlements, communication and dissemination activities.
Sustainable Cognitive Buildings (Franco D. Cicirelli)
Cognitive Buildings (CBs) are entities able to show intelligent capabilities like self-learning, self-managing, and self-adaptation. They need to continuously process environmental parameters and control heterogeneous devices. Sustainable CBs promote sustainability in different forms, e.g., by applying energy-saving policies and by favoring the exploitation of green energy sources. This talk will focus on important aspects of CBs, like architectural approaches, lessons learned in prototyping activities, and platforms for CBs.
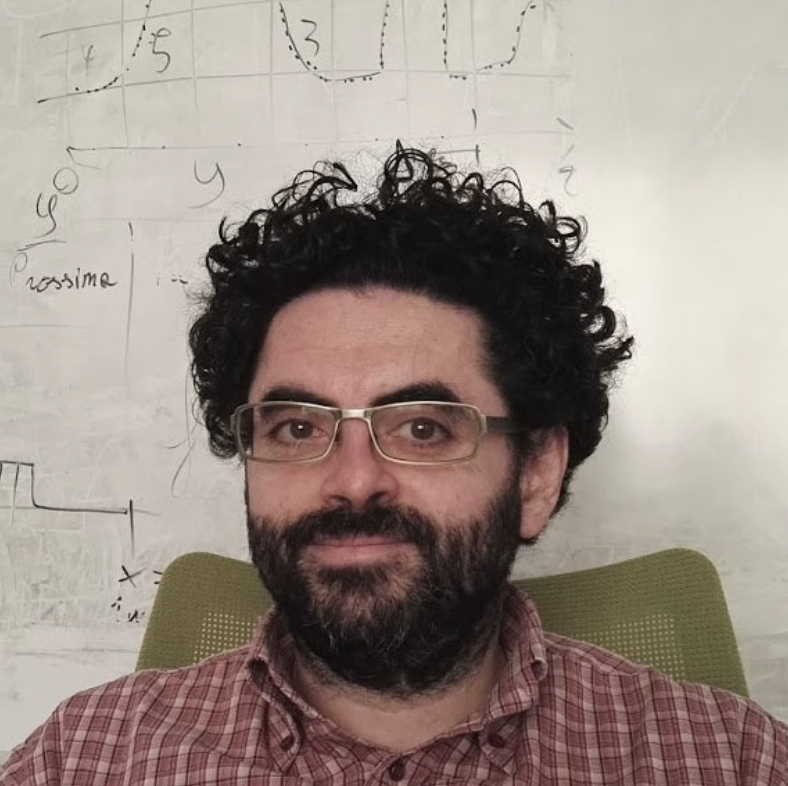
Franco D. Cicirelli is a Researcher at ICAR-CNR since 2015. His research focuses on software engineering tools for modeling, analysis, and implementation of complex distributed systems with temporal constraints (Cognitive Systems and Artificial Intelligence, Internet of Things and Cyber-Physical Systems, edge/cloud continuum, simulation and model checking, formal languages, real-time systems). From 2000 to 2003, he worked at the Research and Development department of the international company TXT e-Solution S.p.a. (Italy). He earned his Ph.D. in Computer Science in 2006. He was a research fellow from 2006 to 2015 at the Department of Computer Engineering, Modeling, Electronics, and Systems (DIMES) of UNICAL. He is an adjunct professor for the Object-Oriented Programming course (DIMES-UNICAL) and previously for “The Fundamentals of Computer Science”; courses (DIMES-UNICAL). He is a co-author of 130 peer-reviewed international articles indexed by SCOPUS, including 34 articles in international journals. He is a member of the program committee of many international conferences and a reviewer for many international journals (IEEE, Elsevier, ACM, MDPI, Inderscience). He has edited books and Special Issues in international venues (Springer, IEEE, MDPI, Inderscience) on topics related to IoT, Urban Ecosystems, Monitoring and Control,
Methodology and Application of Modeling and Simulation, Artificial Intelligence. He is a member of the editorial board of the Int. J. of Simulation and Process Modeling (Inderscience), Modeling (MDPI), Electronics (MDPI), Frontiers in Artificial Intelligence,Frontiers in Internet of Things journals.
Cybersecurity, privacy, bias, and trust in female-oriented technologies. (Maryam Mehrnezhad)
In this talk, we explore the complex risks and harms associated with digital health tech, more specifically, female-oriented technologies (FemTech). FemTech is a range of products such as apps, websites, and IoT devices that focus on the physical, sexual, and reproductive health of the female body. We look at the comprehensive and interdisciplinary methods that are applied to explore the cybersecurity, privacy, trust and bias issues in FemTech. They include the study of the ecosystem (hardware, software, data flow, big data, AI/ML, human dimension, and legal aspects) via the analysis of the related law, and system and user studies.
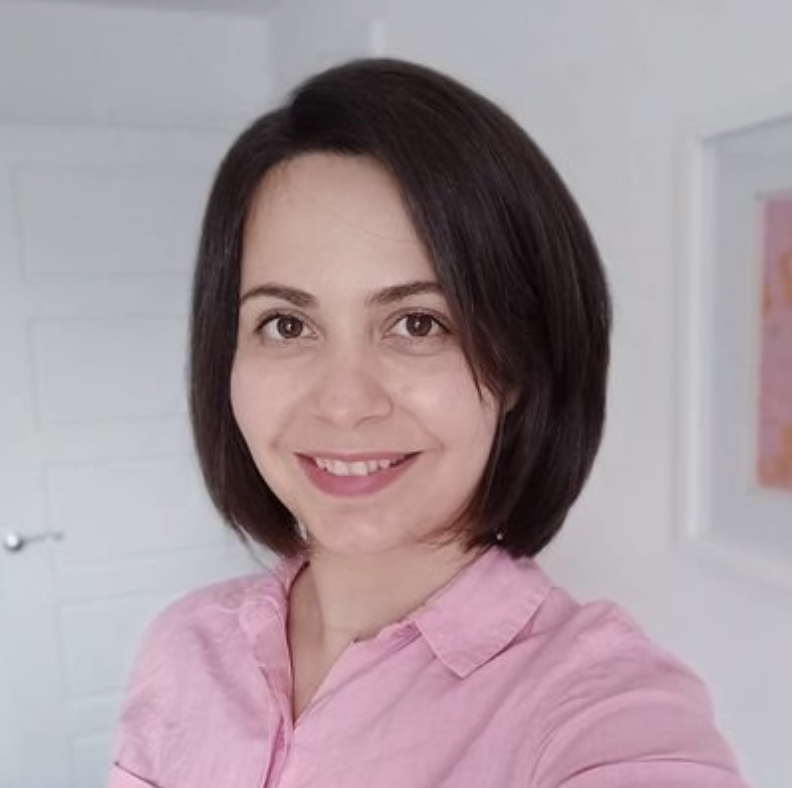
Dr Maryam Mehrnezhad is an Associate Professor at Information Security Department, Royal Holloway University of London (RHUL), UK. Her field of expertise is System Security and Engineering where her research projects are informed by real-world problems. She is the PI of UKRI EPSRC PETRAS CyFer project, and the Co-I of the UKRI EPSRC AGENCY project where she is focusing on the security and privacy of marginalized user groups such as women and girls and people with disabilities.
Analyzing health data: from genomic to clinical data (Antinisca Di Marco)
Analyzing health data is of paramount importance to reduce time to diagnosis and to prescript the right therapy. To address the challenge of precise medicine, in fact information of the health status of a patient at different levels of granularity must be considered at once. New technologies (such as LLM) and the speed-up of computer platforms enable an effective approach to this challenge. In this tutorial, we start discussing open problems and to outline a solution that combines different analysis techniques coming from bioinformatics and AI disciplines in a software engineering flavor.
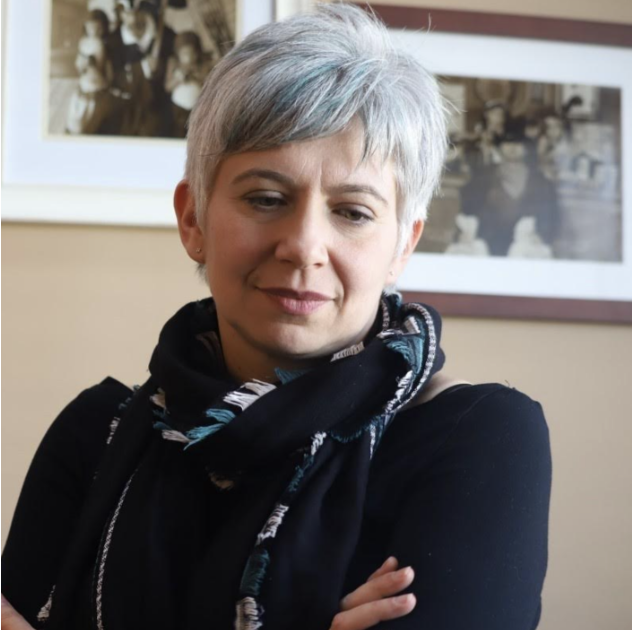
Antinisca Di Marco is Associate Professor in Computer Science at University of L’Aquila. Her main research topics are Software Quality Engineering, Data Science, Quality in Learning Systems and Bioinformatics. She is involved in several national and international projects on such topics. She is responsible for the research infrastructure of the Territori Aperti project, co-PI of the SoBigData.it project and the director of the INFOLIFE CINI Laboratory node in L’Aquila.
Since 2018, she has been involved in several actions and projects aiming at improving equal opportunities in STEM (Science, Technology, Engineering and Mathematics). In particular, she is member of the cost action EUGAIN, and co-ideator and co-coordinator of PinKamP.
Tracking Women’s Health: A Method for Auditing Menopause App Infrastructures (Jennifer Pybus)
Since the pandemic, FemTech, a wide umbrella of women’s health apps, devices, and sensors, has undergone rapid expansion. As use of these technologies increases, so does the datafication of women’s bodies, exacerbating by already-entrenched gendered health discrimination. This talk presents a novel methodology to audit the backend infrastructures of menopause ‘FemTech’ mobile applications, calling attention to the ways in which exceptionally intimate and sensitive health data are being monetised for profit. While apps are supposed to adhere to data protection regulations, their embedded infrastructure is complex, constituted by platforms and third parties who provide proprietary software in a data-for-service economic model. Consequently, this creates blindspots for regulators and policymakers. The mixed methodology we have developed is aimed at addressing this opacity challenge, stemming from the question: how can we effectively audit mobile applications? This involves: i) assessing manifest files; ii) examining software development kits (SDKs); and iii) a qualitative assessment of the apps’ Google Data Safety agreements and privacy policies. Our findings demonstrate the ease with which this data can be accessed from these applications is alarming, especially given that almost every app in our study was sharing email addresses, often alongside user IDs, device identifiers and IP addresses. The analysis is revealing of a troubling lack of clarity for women and folks who identify as women in making informed decisions about how their health data is being shared, signalling a clear need for better regulation and tools to help people make informed decisions about which menopause app to use.
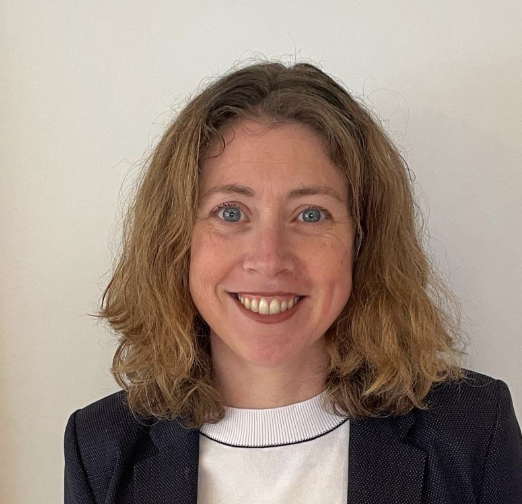
Jennifer Pybus is a globally recognized scholar whose interdisciplinary research intersects digital and algorithmic cultures and explores the capture and processing of personal data. Her work focuses on the political economy of social media platforms, display ad economies, and the rise of third parties embedded in the mobile ecosystem which are facilitating algorithmic profiling, monetisation, polarisation and bias. Her research contributes to an emerging field, mapping out datafication, a process that is rendering our social, cultural, and political lives into productive data for machine learning and algorithmic decision-making.
Resource-Aware Machine Learning (Katharina Morik)
AI today is ubiquitous. And it uses a tremendous amount of energy. Hence, the energy consumption and CO2 footprint of AI should be reduced. This needs to take the hardware into account. Machine learning today needs to make the resource demands of learning and inference meet the resource constraints of the used computer architecture and platforms. A large variety of algorithms for the same learning method and diverse implementations of an algorithm for particular computing architectures optimize learning with respect to resource efficiency while keeping some guarantees of accuracy. Pruning and quantization are ways of reducing the resource requirements by either compressing or approximating the model. For the users it is important to know the trade-off of energy consumption and performance. They may then select the model that best fits their particular application. Trustworthy testing and reporting is needed. Some examples will illustrate easy-to-understand reports of model characteristics for a better model selection.
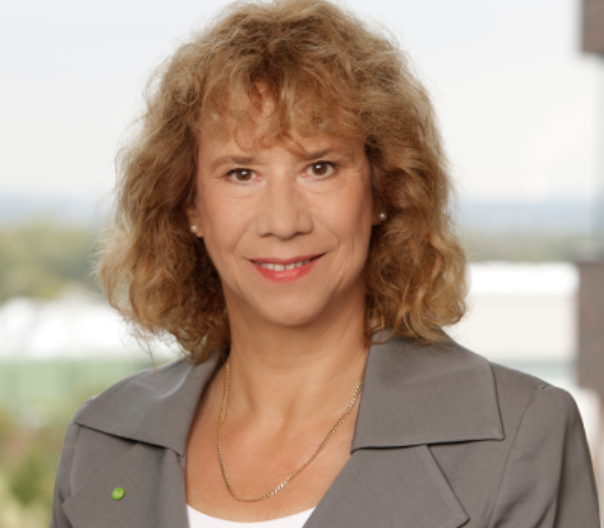
After completing her doctorate in 1981 at the University of Hamburg on belief systems of artificial intelligence, Katharina Morik worked in the project that developed the Hamburg application-oriented natural language system HAM-ANS at the University of Hamburg. At the TU Berlin she took over the internal project management for the joint project LERNER in 1985, in which the first German knowledge acquisition system integrating machine learning was developed. After her habilitation at the TU Berlin (1988), she continued her focus on designing such a system as an assistant to the knowledge engineer (sloppy modeling) within the ESPRIT project “Machine Learning Toolbox” at the Society for Mathematics and Data Processing in St. Augustin. In 1991 she accepted the call for the C4 professorship in the Department of Computer Science at the University of Dortmund.